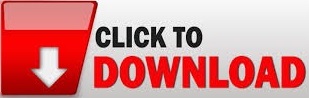
They achieved this by fine-tuning an ImageNet CNN to predict a much wider set of visual concepts (visual n-grams) from the text of titles, descriptions, and tags of 30 million Flickr photos and were able to reach 11.5% accuracy on ImageNet zero-shot.įinally, CLIP is part of a group of papers revisiting learning visual representations from natural language supervision in the past year. Most inspirational for CLIP is the work of Ang Li and his co-authors at FAIR who in 2016 demonstrated using natural language supervision to enable zero-shot transfer to several existing computer vision classification datasets, such as the canonical ImageNet dataset. The same year DeVISE scaled this approach and demonstrated that it was possible to fine-tune an ImageNet model so that it could generalize to correctly predicting objects outside the original 1000 training set. In 2013, Richer Socher and co-authors at Stanford developed a proof of concept by training a model on CIFAR-10 to make predictions in a word vector embedding space and showed this model could predict two unseen classes. A critical insight was to leverage natural language as a flexible prediction space to enable generalization and transfer. The idea of zero-data learning dates back over a decade but until recently was mostly studied in computer vision as a way of generalizing to unseen object categories. This is a key change: by not directly optimizing for the benchmark, we show that it becomes much more representative: our system closes this “robustness gap” by up to 75% while matching the performance of the original ResNet-50 on ImageNet zero-shot without using any of the original 1.28M labeled examples.ĬLIP ( Contrastive Language–Image Pre-training) builds on a large body of work on zero-shot transfer, natural language supervision, and multimodal learning. By design, the network can be instructed in natural language to perform a great variety of classification benchmarks, without directly optimizing for the benchmark’s performance, similar to the “ zero-shot” capabilities of GPT-2 and GPT-3. We present a neural network that aims to address these problems: it is trained on a wide variety of images with a wide variety of natural language supervision that’s abundantly available on the internet.
Benchmark testing clip art update#
Update your Dockerfile with the following.Although deep learning has revolutionized computer vision, current approaches have several major problems: typical vision datasets are labor intensive and costly to create while teaching only a narrow set of visual concepts standard vision models are good at one task and one task only, and require significant effort to adapt to a new task and models that perform well on benchmarks have disappointingly poor performance on stress tests, casting doubt on the entire deep learning approach to computer vision. When using the RUN statement, our tests run when building the image, and stop the build when they fail. The CMD statement is not executed during the building of the image, but is executed when you run the image in a container. We can improve this slightly by using a RUN statement instead of the CMD statement in the test stage. However, we'll have to run two Docker commands to build and run our tests. The build output is truncated, but you can see that the Maven test runner was successful and all our tests passed. Tests run: 40, Failures: 0, Errors: 0, Skipped: 1 $ docker run -it -rm -name springboot-test java-docker
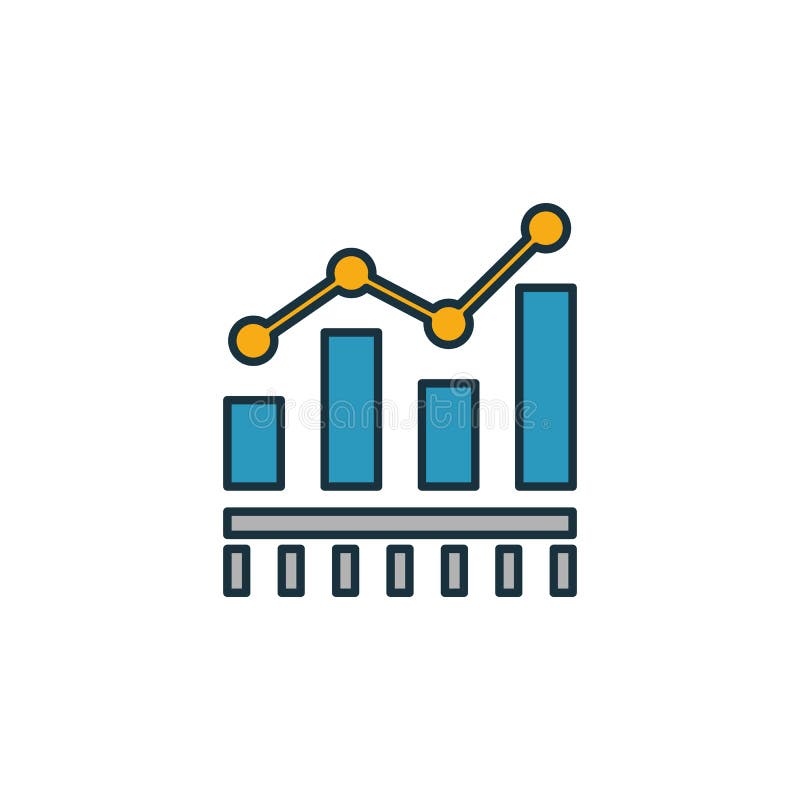
Best practices for Dockerfile instructions.
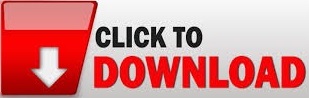